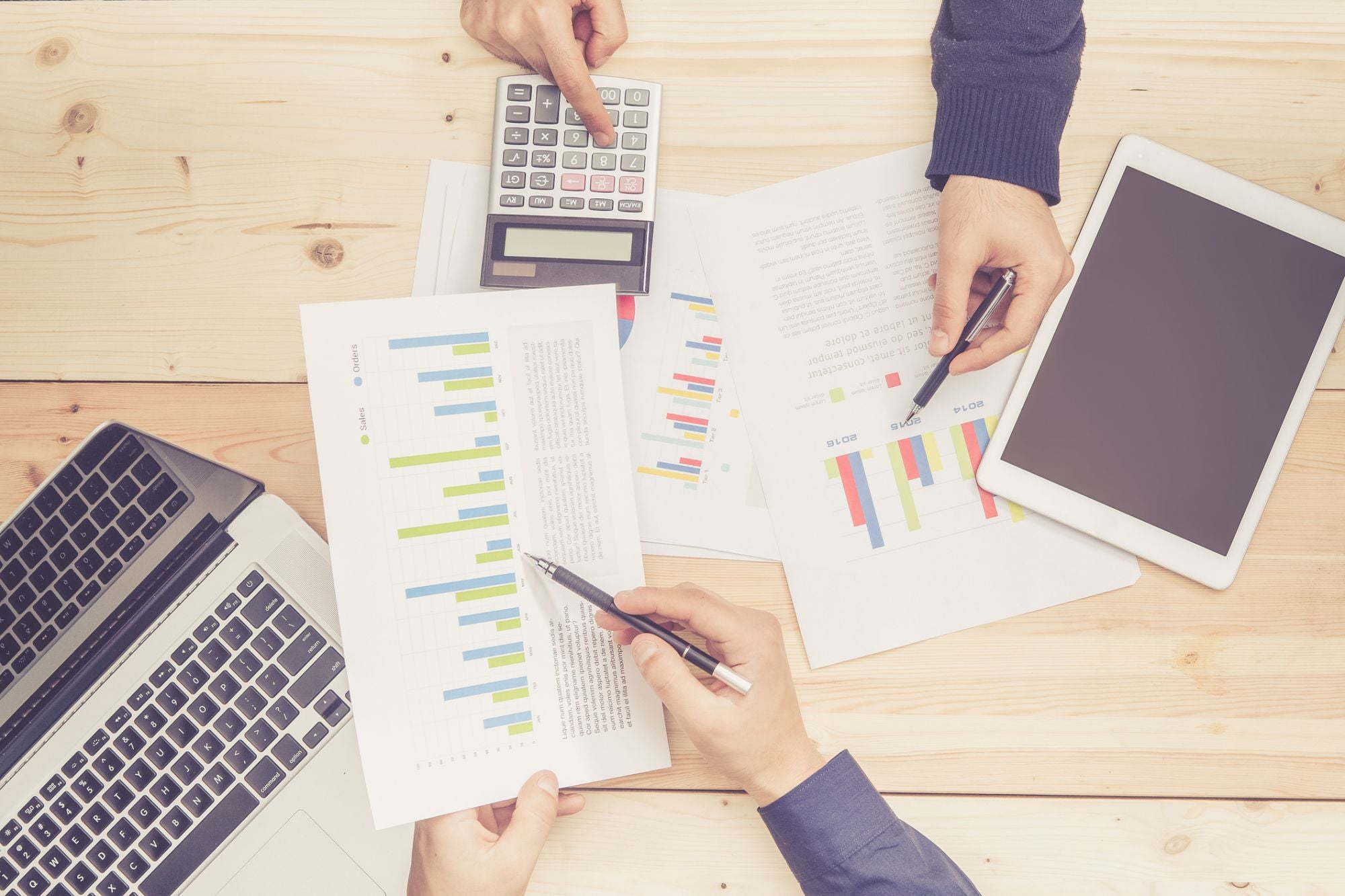
If you’re not combining your tests with personalization efforts, you’re doing it wrong.
6 min read
Opinions expressed by Entrepreneur contributors are their own.
Successful startups today try new things quickly. Founders don’t spend years investing time and money to build a business only to find the market doesn’t want the product or the model doesn’t scale. Smart entrepreneurs fail fast. They limit the damage and move on to the next potentially winning idea.
This mentality is prevalent in marketing, too, due primarily to A/B testing. The practice has taken much of the guesswork out of marketing, helping transform it into one of the most measurable business-development disciplines. But the way marketers have approached A/B testing over the years doesn’t cut it in today’s world. Here’s why, and how you can fix it.
What is A/B testing?
An internal debate springs up whenever a business takes on a new marketing initiative. This headline or that one? This subject line or that one? This image or that one? Each team member has an opinion. In the past, groups needed to reach agreement. Members could select only one option for each element, and achieving that consensus often took time.
A/B testing changed the dynamic. You no longer must commit to one version of anything — you can test a few different approaches in small batches. An A/B test displays two (or more) experiences to your audience so you can measure the impact of each and statistically determine which was most successful.
Related: 6 Reasons Why Attracting the Right Online Traffic Is Your Top Priority
Imagine you plan to send an email, and you’re torn between two subject lines. A/B testing enables you to assign a Subject Line A and a Subject Line B to an initial, smaller list of recipients. You’ll want to answer questions such as:
- Which had a higher open rate?
- Which had a higher clickthrough rate?
- Which ultimately drove more conversions?
Obviously, you’ll use the winner when you send the rest of the messages to the larger list of remaining email addresses. You can apply the same approach to test different home page experiences, calls to action (CTAs), ad copy, blog titles and other components. Each test helps you refine your strategy for future efforts.
This data-driven model clearly improves on the guessing method. But while A/B testing has done much for the marketing discipline, it doesn’t go far enough on its own to meet the needs of modern marketing professionals. It’s one tool in your kit.
A/B testing can get better.
A/B testing has one major limitation: It tells you only which experience works best for the majority. That sounds like a win at first. But this mindset risks ignoring a group of people for whom the experience doesn’t work at all. These users might be annoyed or confused by the experience, and they can be a very vocal minority.
In the past, marketers considered this to be an acceptable gamble. After all, shouldn’t you want the message that resonates with the most people?
Consider all the A/B testing that goes into determining a website’s home page. The marketing team designs several different versions. Members test each version to determine which works best. Then, logically, the team pushes out the winning experience to 100 percent of visitors. But they continue to tweak the home page by testing different headlines, CTA button colors or sizing and content promotions.
You don’t need to pick just one winning layout, image, headline or CTA. You can select the right one based on all that you know about a person.
Related: How to Test Different Versions of Your Website in 6 Easy Steps
How to improve A/B testing.
You still can benefit from A/B testing in a personalized world. The key is to stop thinking about A/B testing solely in a one-size-fits-all sort of way. Instead, work toward combining your No. 1 finisher with personalization efforts. That’s how you find the winning experience for each person.
Savvy marketers think about A/B testing with both types of personalization experiences: segments (groups of people) and individuals (one-to-one). Here’s how.
Segment experiences.
Segment-level personalization tailors an experience to a group of people based on shared characteristics. For example, you could send one email promotion to shoppers interested in shoes and a different promotion to those interested in sweaters. Your homepage could display one headline to visitors from small businesses and a different headline to users from large enterprises. You could deliver one message to new visitors and one to returning visitors, or you could roll out one experience to prospects and another to existing customers. You get the idea.
Rather than testing different generic versions of the home page to find the one experience that works best for most people, you can test different versions of the homepage tailored to each of your target audiences.
Related: 2 Simple Rules to Follow When Developing a Market Segmentation
Individualized experiences.
Individualized experiences take the idea a step further. Machine-learning algorithms make it possible to evaluate everything known about a person and select the experience that’s most relevant for him or her. You’ve no doubt seen this at work in product or content recommendations, but it also can be used to suggest categories or brands. Other applications include list-sorting and navigation-ordering.
Combining this type of one-to-one personalization with A/B testing actually seeks to test the algorithm itself. A good personalization solution will allow you to control the algorithms.
Related: Netflix-like Recommendations May Be in Store for Workplace Benefits
Imagine you work for a retail e-commerce business that seeks to launch product recommendations on its home page. You’d be wise to test a few different versions of the algorithm driving those recommendations. Should the page display new products? Products the visitor has browsed before but didn’t buy? Trending products? Or perhaps it should advance items the visitor is most likely to enjoy based on her or his preferences for brands, categories, price range or some other trait.
Trialing a few variations on the algorithm allows you to find the best option. You can even test different algorithms for different audiences. Maybe one algorithm performs best for new visitors while another performs best for returning visitors.
Comments are Closed